Dynamics-Regulated Kinematic Policy
for Egocentric Pose Estimation
Zhengyi Luo, Ryo Hachiuma, Ye Yuan, Kris M. Kitani
NeurIPS 2021
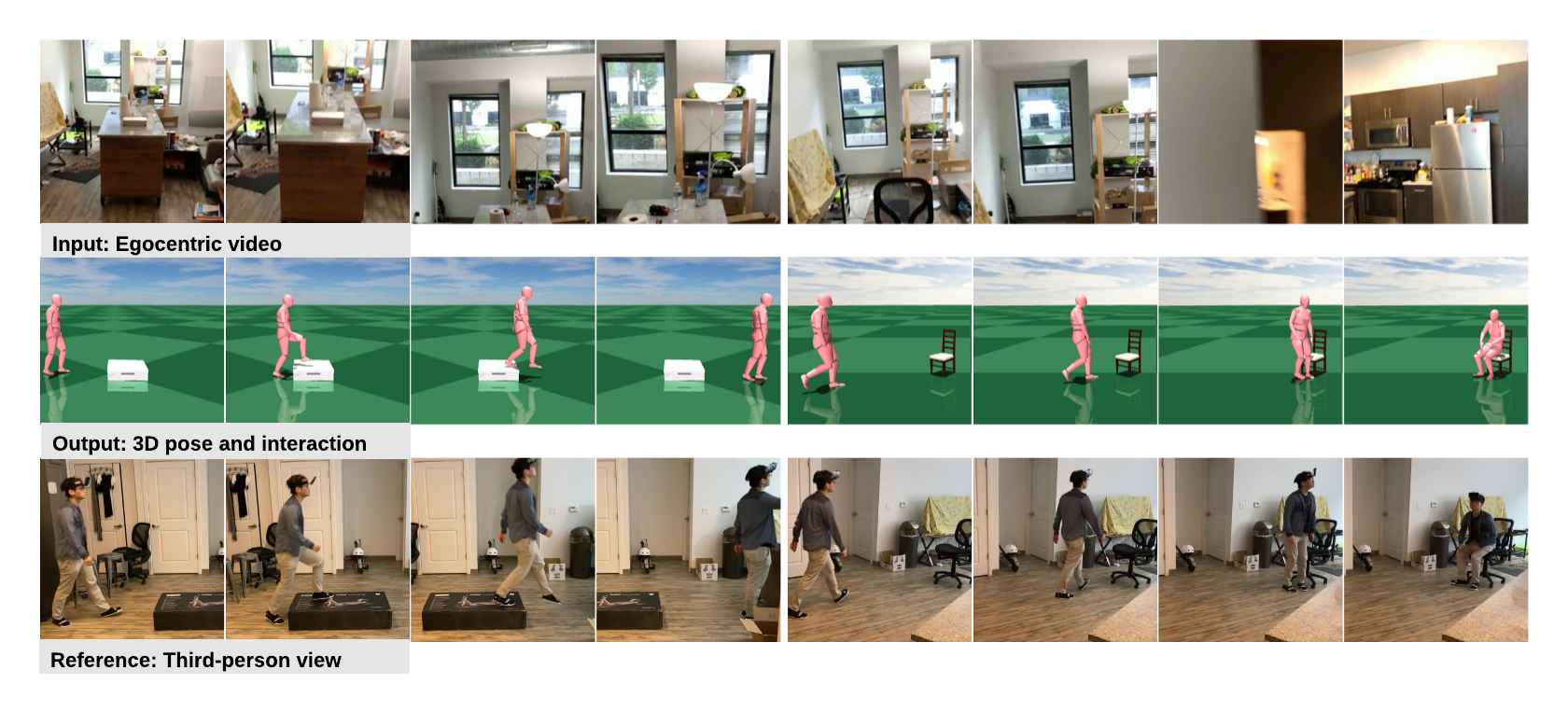
We propose a method for object-aware 3D egocentric pose estimation that tightly integrates kinematics modeling, dynamics modeling, and scene object information. Unlike prior kinematics or dynamics-based approaches where the two components are used disjointly, we synergize the two approaches via dynamics-regulated training. At each timestep, a kinematic model is used to provide a target pose using video evidence and simulation state. Then, a prelearned dynamics model attempts to mimic the kinematic pose in a physics simulator. By comparing the pose instructed by the kinematic model against the pose generated by the dynamics model, we can use their misalignment to further improve the kinematic model. By factoring in the 6DoF pose of objects (e.g., chairs, boxes) in the scene, we demonstrate for the first time, the ability to estimate physically-plausible 3D human-object interactions using a single wearable camera. We evaluate our egocentric pose estimation method in both controlled laboratory settings and real-world scenarios.
Overview
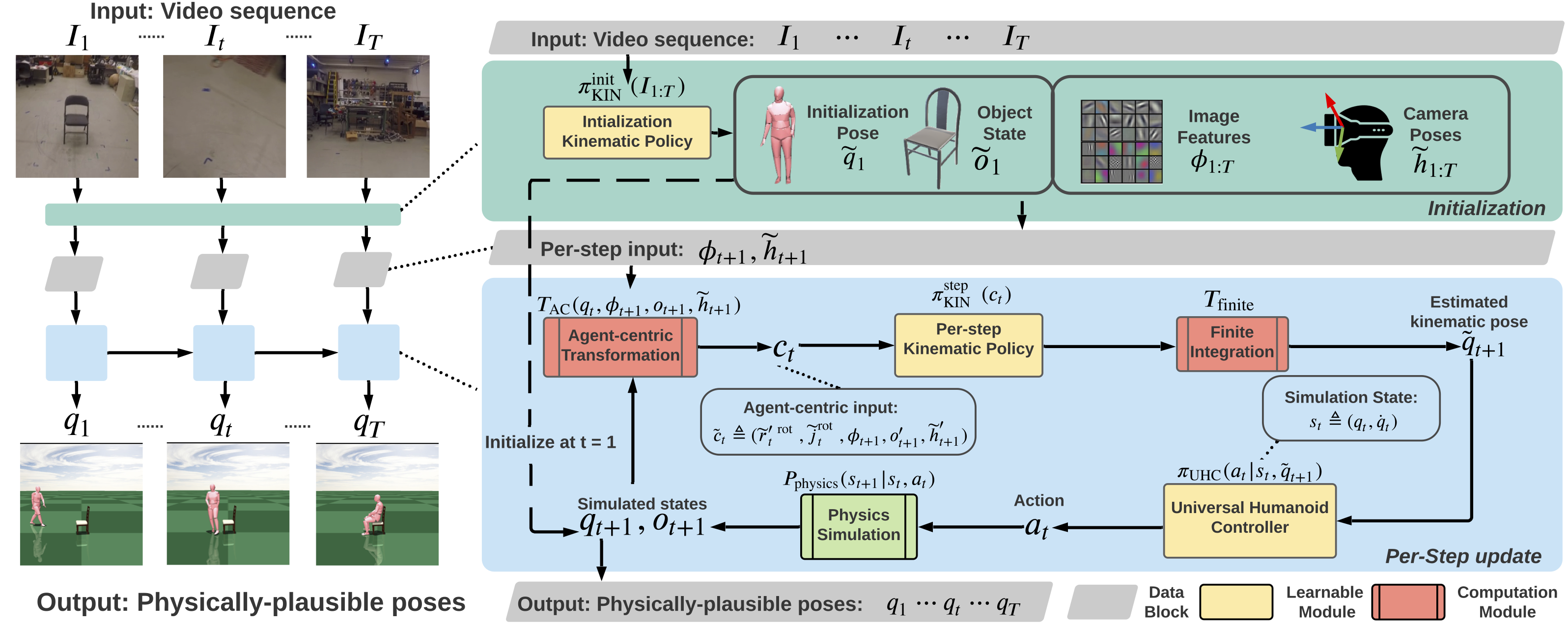
Our framework first learns a Universal Humanoid Controller (UHC) from a large MoCap dataset. The learned UHC can be viewed as providing the lower level muscle skills of a real human, trained from mimicking thousands of human motion sequences. Using the trained UHC, we learn our kinematic policy through dynamics-regulated training. The kinematic policy provides per-step target motion to the UHC, forming a closed-loop system that operates inside the physics simulation to control a humanoid. For more details, please checkout our paper and supplementary video:
10 mins talk
Demo Video
Paper and Code
--